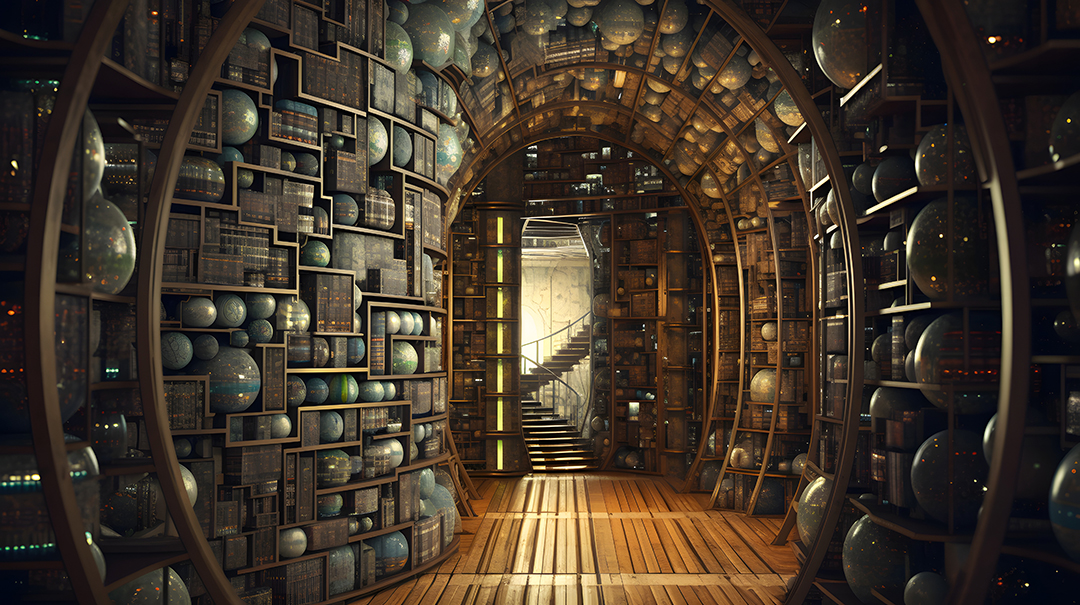
How AI Is Rewiring Language Quality Management Workflows
Managers and leaders throughout the localization industry are grappling with the impact of AI. The same goes for the language quality professionals who make translation and localization more effective.
What does AI mean for everyday language quality management? Machines aren’t likely to replace language quality reviewers and subject-matter experts anytime soon.
Instead, AI is rewiring what people do and how they perform their jobs. Just as machine translation has changed the process of localization, generative AI will speed up tasks and streamline quality assurance and control.
Let’s explore what’s possible right now, what benefits and risks come with growing automation, and how machine intelligence will likely change future language quality workflows.
The Future of Language Quality Is AI-Assisted
Today, AI is already working its way into off-the-shelf quality review tools, making various tasks faster and easier.
- Classifying and rating errors: AI can categorize linguistic mistakes, rate their severity, and explain the reasons behind the ratings.
- Highlighting problem areas: AI-powered software can assess the quality of text segments, telling reviewers which sections are error-free and which need a closer look.
- Streamlining project management: AI project orchestration tools can decide when to import content for review, saving project managers time so they can concentrate on other important tasks.
These current tools only go so far. For example, humans are still fully responsible for identifying linguistic errors.
Nonetheless, the technology is sure to keep improving. As time goes on, AI support will become more routine and enmeshed in everyday language quality workflows. That means fewer repetitive tasks and faster, quicker solutions.
Deeper AI involvement brings benefits, tradeoffs, and risks
While off-the-shelf tools are advancing, custom AI could provide even more advanced assistance for language quality reviewers. For example, a company could train an LLM to spot deviations from its brand voice or maintain consistent terminology specific to the organization.
Nevertheless, there are tradeoffs to consider for any organization contemplating this route.
- A custom AI model trained on internal data maximizes privacy and control. On the downside, custom development is costly and time-consuming.
- Using a third-party API like OpenAI could be a quicker and cheaper option. However, it means giving your company’s data to an external provider. You should assess what data you’re comfortable sharing and the risks involved. Think twice about handing sensitive information over to outside AI platforms, as you might not know how they use your data.
- Off-the-shelf tools offer the least advanced capabilities but also the lowest costs. If your organization has less specialized needs and fewer resources, it may make sense to stick with what’s available out of the box.
Does it make sense to entrust language quality reviews entirely to a machine-learning model?
At the moment, creating such a system would require a major investment of time and resources. It could raise data security concerns if you develop it by integrating your TMS with a third-party platform such as OpenAI.
Above all, today’s most advanced LLMs still miss many errors and nuances that a human reviewer would catch. That makes it a risky choice if your localization program requires even a modest level of linguistic quality. You need a human eye in the mix, regardless of your strategy.
How human-machine collaboration is evolving
Because of these limitations, the current wave of AI is unlikely to remove humans from the loop. Instead, humans are working more and more closely with machines.
So, where is this trend leading? Let’s examine what we could see next, with the caveat that no one knows precisely what new changes are waiting around the corner.
AI will take on more responsibilities.
AI-driven systems can train on feedback from quality reviewers to learn what grammatical or syntactical errors to flag and how to handle more refined challenges such as idioms, tone, or style. With models trained on high-quality data, language quality management teams will eventually be able to trust AI with more varied and complex tasks.
The human role will shift in focus.
As AI gains the ability to detect technical linguistic errors, human quality reviewers will likely focus more on addressing linguistic nuances such as style and tone.
Just as machine translation has turned translators into post-editors, language quality professionals may become “post-reviewers”, vetting the accuracy of AI-driven quality checks. Along the way, their feedback will help train and optimize AI systems to catch more errors over time.
AI-generated content will further transform language quality workflows.
For various use cases, AI will allow companies to skip translation and directly generate content in target languages.
Such workflows may bring language quality experts into the process at an earlier stage, working directly with content creators and developers to ensure high-quality outputs. Generative AI can often create content that sounds plausible but is simply wrong—so language quality reviewers may also take on a new role as fact-checkers.
Regardless of what happens, humans will play an essential role in oversight.
Human experts bring skills that artificial systems can’t match, such as judgment, critical thinking, and contextual understanding.
In addition, high-quality training data is lacking for many languages besides the most widely spoken ones (such as English). For all these reasons, AI will probably take some time to catch up with human linguistic diversity.
For all these reasons, human reviewers will need to stay involved to ensure the final product is up to standard, even if AI handles more of the routine work. They will keep doing what machines can’t naturally do on their own: adapt and evolve to an ever-changing environment.
What’s the Big Picture for Language Quality Management?
Today, AI is only getting started.
For now, most AI-powered tools assist human quality reviewers at the margins. However, machine intelligence is poised to have a more profound impact on language quality workflows as more capable systems become available.
In the near to medium term, language quality managers will likely need to experiment with different options and answer many questions case by case. For example:
- Which aspects of language quality review are cost-effective and feasible to automate, given the benefits, costs, and resources available?
- What’s the right balance between AI and human expertise? How should an organization weigh the efficiency gains of AI-assisted workflows against the risks of too much automation?
- What’s the optimal workflow for improving the quality of AI-generated content?
Whatever the future holds, the need for effective language quality management is unlikely to go away.
On the contrary, generative AI is set to flood the world with content in a wider range of languages than ever. Localization and language quality leaders will have to put more thought into creating workflows that can ensure linguistic quality at scale.
Are you looking for an edge in the era of AI? Contact Beyont to explore how language quality management can boost your localization program.